Split lets companies fulfill the promise of continuous delivery: with Split’s safe launch and experimentation, teams can take any feature into production with fine-grain control. In order to precisely release features (and gather data on their engagement), Split’s SDKs utilize different streams of data. And many of our customers run Split with SDKs installed in multiple languages and throughout their application stack. By default, Split’s SDKs keep Split segment and Split targeting data synchronized as your users navigate across large distributed systems, treatments and conditions. Some languages, however, do not have a native capability to keep a shared local cache of this data to properly serve treatments.
Today, we’re giving these languages the power to do so by introducing a new feature: the Split Synchronizer. Let’s take a look at how it works, and the performance boost it can give your app in serving treatments with Split.
How the Split Synchronizer Works
The Split Synchronizer coordinates the sending and receiving of data to a remote data store that all of your processes can share when updating definitions and rules for the evaluation of treatments. Its an easy-to-install job that sits on your servers and is designed to run entirely in the background so that it has zero impact on the performance of your application servers,. Split supports Redis as a remote data store out-of-the box, so it’s no surprise the Split Synchronizer utilizes Redis as the cache for your SDKs when evaluating treatments. It also posts impression data and metrics generated by the SDKs back to Split’s servers, for exposure in the web console or sending to the data integration of your choice.
The Split Synchronizer works with most of the languages Split supports:
Those not currently listed already have the ability to keep a local data cache for Split’s SDKs, but if you’re already a Split customer and curious about using the Synchronizer with a language not listed above, drop us a note.
Benefits
Why take the Synchronizer approach? Whether you’re working with one Split SDK or many, your team will see the benefits of:
- Data Consistency: Split-synchronizer and associated SDKs implement a producer-consumer pattern, using a Redis cache as a remote data store to guarantee data consistency. This allows you to take ownership of the data store, giving your team the power to improve the performance when feature flag definitions are requested from the SDK.
- Security: Since the Redis instance is located on either your local network or behind a virtual private network (VPN), any data contained within your features flags will remain in your app and secure.
- Fault Tolerance: If for some reason a connection issue is experienced on your network, the last consistent state of your feature flags will be stored in the Redis cache, and the Split Synchronizer will synchronize it once that the connection issue is restored.
- Error Handling: In addition to logging errors in the file system or even in the stdout, Split Synchronizer has the ability to send errors to a customized Slack channel or Slack user.
Service Architecture
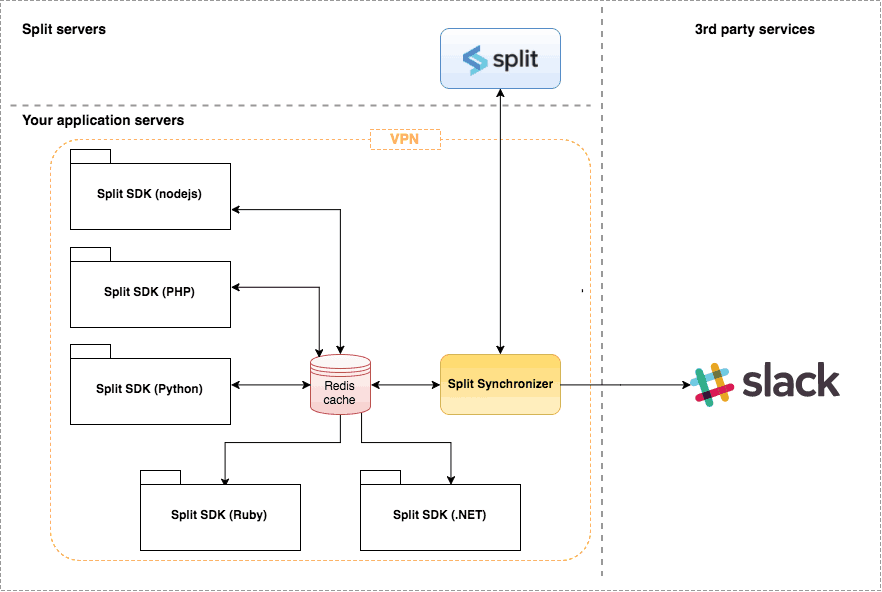
This service performs four different actions:
- Fetch Splits: retrieve the split definitions.
- Fetch Segments: retrieve your segments lists and memberships to targeting groups.
- Post Impressions: send the impressions generated by the SDK to Split servers.
- Post Metrics: send different metrics (like latencies) from the SDK to Split servers.
Performance Improvements and Benchmark
The Split Synchronizer is written in Go and introduces notable performance improvements over previous language-specific synchronizers bundled with our SDKs. Now, tracked data (Split’s impressions log) will be posted at Split servers in a near real-time window.
The following numbers show an SDK’s impression-handling capability over time, after installing the Split Synchronizer:
~3M /minute | ~180M /hour | ~4.32B /day |
These numbers belong to the test scenario below:
Spliti-sync instance | m4.large (2 CPU — 8 GB Memory) |
Redis instance | cache.m3.large (2 vCPU — 6 GB Memory) |
Number of features flags | 200 |
Impressions to send per post | 500k |
Impressions generated per feature flag | 100k |
Insertion rate in Redis | 1M each ~5s |
Duration of the benchmark | 48 hours |
Analyzing Performance Improvements
Let’s look at performance by analyzing some infrastructure numbers for the duration of this benchmark .
The rate of successfully posted impressions remained stable throughout the duration of the test:
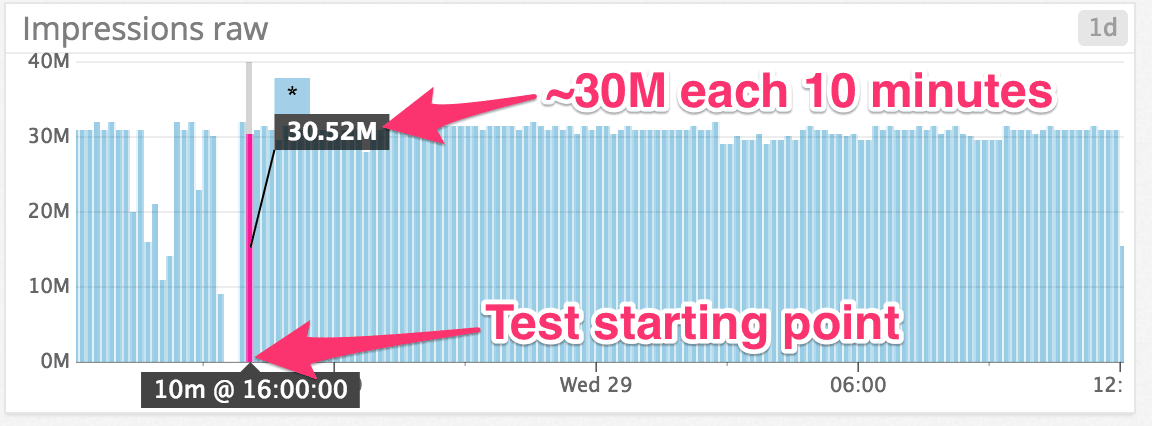
Memory consumption remained stable during the benchmark:

The CPU remained constant and within boundaries (~25% to 55%):
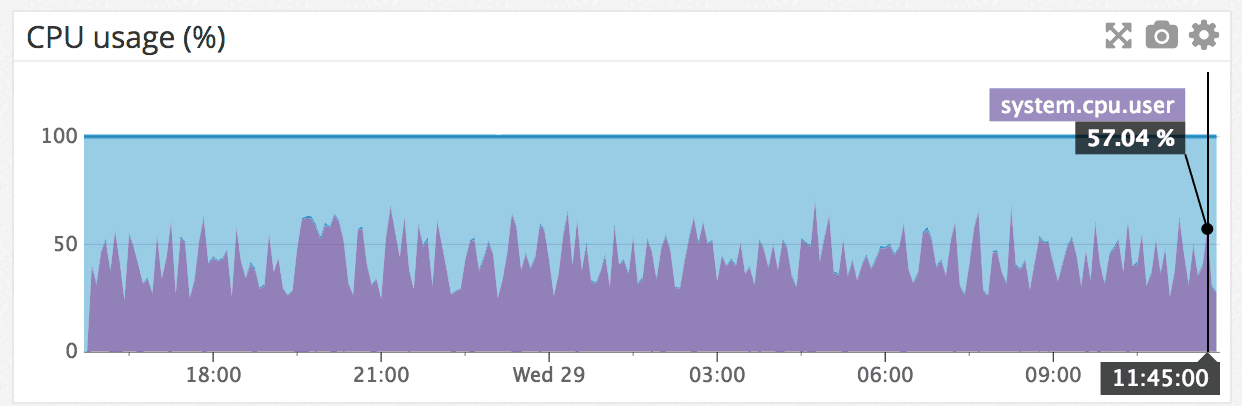
Throughput remained constant at ~20MB/s:
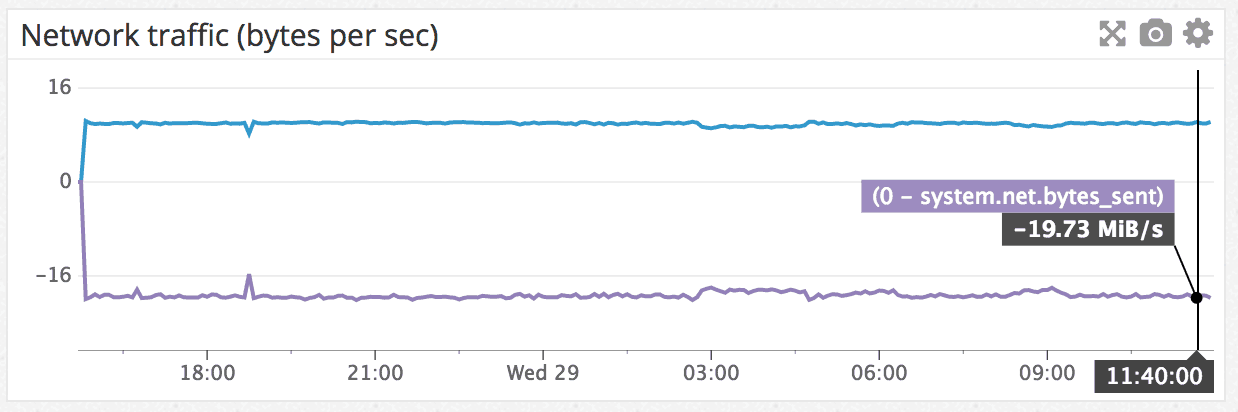
Want to Dive Deeper?
We used a lot of technical terms in this blog, so see definitions below.
What Are Feature Flags?
Feature flags, also known release toggles, or permission toggles, are a software development process that allows developers deploy features in a feature release to the production environment without showing them to users. This essentially decouples deploy from release. They also enable or disable certain features in runtime through a kill switch. Feature flags are typically used to control the rollout and optimization of new features, experiment with different functionalities, or manage the deployment of code changes without rollbacks, debugging, hurting the codebase or the user experience. Use cases for feature flags include canary releases, trunk-based development, CI/CD, Continuous Delivery. There are a lot of providers, but here at Split, we are partial to Split 🙂
Then What Are Feature Toggles?
Feature toggles are just another name for feature flags. The concept was originally coined by Martin Fowler and Jez Humble. They popularized the idea of the separation of feature rollout from code deployment.
In our glossary we define a feature toggle as a snippet of code that tells a feature to activate or deactivate in a given code base. This can be done using low tech methods like configuration files to high-tech methods such as a holistic feature flag management system to manage every toggle configuration in one platform (like Split).
How Do Feature Flags or Feature Toggles Differ From Feature Branches?
Feature branches are a software development approach where each new feature is developed in its own feature branch separate from the main code line currently in production.
A feature flag is a toggling feature used in CI/CD (continuous integration/continuous delivery) environments that allows for new features to be deployed directly to the main code line in a disabled state.
What Is A/B Testing?
A/B testing is the approach of testing two different versions of a web page or product feature in order to optimize conversion rate, or improve upon a certain business metric. For a full definition, see our glossary page.
Split’s Developer Hub
Visit our Developer Hub to find content like our Guide to APIs: REST, SOAP, GraphQL, and gRPC.
Switch It On With Split
The Split Feature Data Platform™ gives development teams the confidence to move fast without breaking things. Set up feature flags and safely deploy to production, controlling who sees which features and when. Connect every flag to contextual data, so you can know if your features are making things better or worse and act without hesitation. Effortlessly conduct feature experiments like A/B tests without slowing down. Whether you’re looking to increase your releases, to decrease your MTTR, or to ignite your dev team without burning them out–Split is both a feature management platform and partnership to revolutionize the way the work gets done. Switch on a free account today, schedule a demo, or contact us for further questions.
Get Split Certified
Split Arcade includes product explainer videos, clickable product tutorials, manipulatable code examples, and interactive challenges.